Artificial Intelligence
Joint Research Initiative
France
Achieving interpretability for big data and machine-learning systems
"The recent controversy in France concerning the opacity of the APB process – an algorithm that assigns students to universities –, is a good example of the need for interpretability when it comes to machine-learning systems", offers Prof. Christophe Marsala. "French students are asking to know how the algorithm sorts through the candidates when the university course they are asking for is saturated. Students are complaining because they don't understand the decision." "In the current context of big data and data science challenges, it appears that, if it is essential to build reliable and efficient systems, it is also crucial to offer interpretable systems and interpretable decisions", the researcher points out.
From machine learning black boxes to interpretable insights
The motivation for this project comes from the observation that defining interpretability is in itself a difficult task to solve and that it constitutes a challenge."Interpretability is concerned with the inner structure of the studied system that can for instance be a mathematical function, a set of rules or a decision tree to name a few. It refers to its validity, its readability, its intuitive coherence or its outputs", Prof. Marsala explains. "Moreover, interpretability is also a highly subjective concept as it depends on whom it is intended for. The interpretation of a model is directly related to its recipient, his expectations and his knowledge" Whether you are the end-user, as is the case with French students and the APB process, a domain expert or a data scientist for instance, your conception of what an interpretable system should look like i completely different.
The AXA Joint Research Initiative intends to take a global view of interpretability by taking into account these different perspectives. More specifically, the project proposes to distinguish between four types of interpretability, reflecting the complexity of the inner structure of such systems and the various expectations. To construct all four interpretability definitions, a cross-disciplinary approach will be applied, in particular combining computer with cognitive sciences. The next step will be to explore methods that intrinsically offer interpretability properties to either be added to existing systems or incorporated in future ones.
The project directly addresses current challenges posed by digital transformation, especially when it comes to big data technologies and embedding Machine Learning in the operational environments. This aspect is of particular importance to AXA and to the insurance sector more generally. Interpretability will be crucial to guarantee customer trust for instance, or to provide regulatory organs with the proof that legal requirements are respected. "The proposed approaches will allow to move from machine learning black boxes to interpretable insights, opening up the possibility to understand and even change decision-makers’ actions", summarises Prof. Marsala. The findings should provide solid foundations for the creation or adaptation of current algorithms to obtain human-friendly versions.
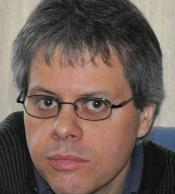
Christophe
MARSALA
Institution
Sorbonne University
Country
France
Nationality
French
Related articles
Artificial Intelligence
AXA Chair
United Kingdom
Explainable AI for healthcare: enabling a revolution
Developing technologies that we can trust: a new paradigm for AI As these limitations have become increasingly apparent, AI experts... Read more
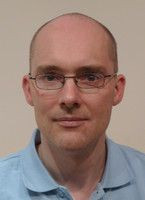
Thomas
LUKASIEWICZ
University of Oxford
Artificial Intelligence
Joint Research Initiative
Belgium
Fairness in AI: ending bias and discrimination
Garbage in, garbage out: how to ensure fairness-aware machine learning? When measuring fairness, a natural preliminary question to ask is... Read more
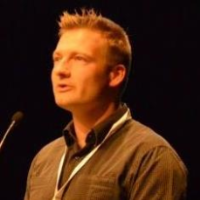
Toon
CALDERS
University of Antwerp
Artificial Intelligence
Joint Research Initiative
Belgium
Fulfilling the potential of AI: towards explainable deep learning
“In its approach of explainable AI, the project will investigate the use of instance-based explanations (explaining the model for one... Read more
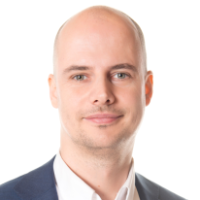
David
MARTENS