Artificial Intelligence
Post-Doctoral Fellowships
Germany
2018.12.31
Fairness in Machine Learning: Algorithmic Discrimination and Exploitation as a Challenge for EU Law
How to adapt the EU legal framework to the challenges of the future
The question Dr. Philipp Hacker asks is, how do we offer people the assurance that the ML algorithms can take ‘fair’ and trustworthy decisions on a legal level? To be able to provide an informed answer, the researcher intends to proceed in consecutive steps, starting with questions about the current doctrinal structure offered by EU law in this context: Are current regulatory strategies efficient? What are their limits? The researcher will then be able to tackle the issue of new regulatory tools to address the shortcomings of the existing ones. This step of the project will draw primarily on technologically-informed regulations. Two main strategies will be investigated: personalized law, and what may be called ‘principled coding rules’. The first uses ML to detect the potential vulnerability of a data subject, in order to then provide specific legal protection; the second infuses regulation directly into algorithms by intervening during the coding process. “Personalized law brings ML technology to regulation; conversely, in principled coding, regulation is infused into coding”, resumes Dr. Hacker. Both strategies have their upsides and downsides, the major one being that the first allows for more flexibility but creates its own problems of privacy protection as regulators, too, gain access to citizen’s data.
Regulation of the digital economy is one of the most pressing and cutting-edge topics of legal research. Dr. Hacker’s research approach innovatively offers to move beyond the current fixation on privacy in the area of Big Data and Machine learning and to address topics that have received too little attention so far, namely discrimination and exploitation. His approach, considering ML both as an object of regulation and as a means, is also very novel. Finally, the simultaneous treatment of both discrimination and exploitations risks holds great promise of cross-fertilization in the design of novel and state-of-the-art regulatory solutions.
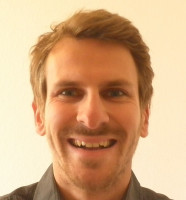
Philipp
HACKER
Institution
Humboldt Universitaet zu Berlin
Country
Germany
Nationality
German
Related articles
Artificial Intelligence
AXA Chair
United Kingdom
2019.09.01
Explainable AI for healthcare: enabling a revolution
Developing technologies that we can trust: a new paradigm for AI As these limitations have become increasingly apparent, AI experts... Read more
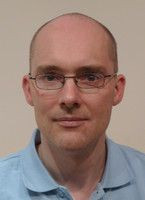
Thomas
LUKASIEWICZ
University of Oxford
Artificial Intelligence
Joint Research Initiative
Belgium
2019.07.30
Fairness in AI: ending bias and discrimination
Garbage in, garbage out: how to ensure fairness-aware machine learning? When measuring fairness, a natural preliminary question to ask is... Read more
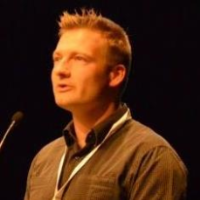
Toon
CALDERS
University of Antwerp
Artificial Intelligence
Joint Research Initiative
Belgium
2019.10.31
Fulfilling the potential of AI: towards explainable deep learning
“In its approach of explainable AI, the project will investigate the use of instance-based explanations (explaining the model for one... Read more
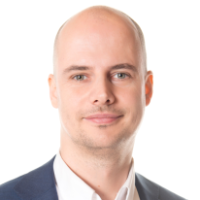
David
MARTENS