Economics
Post-Doctoral Fellowships
Switzerland
2008.12.31
Neural Basis of expected utility and mean variance model of decision making
My research focuses on contrasting expected utility and mean-variance thinking in financial decision making. The impetus for this are recent findings that the human brain appears to encode both approaches. This may suggest that the human brain, through evolutionary pressures, is attempting to make the best use of both mean-variance and expected utility models.
The Probabilistic Brain
Biography
Mathieu d'Acremont was born in France (Nantes) and moved to Switzerland in 1980, when he was seven years old. He started his study in psychology at the University of Lausanne. He received his Master of Advanced Studies in Clinical Psychology (1999) and a certificate in Cognitive Neuroscience (2000) at the University of Geneva. He obtained a PhD in Psychology (2005) and a Master in Statistics (2008) at the same university. After his PhD, Mathieu d'Acremont worked as a senior researcher at the Swiss Center for Affective Sciences in Geneva where he conducted studies on impulsive behavior and decision-making in youth. In 2007, he was invited at the Brain and Creativity Institute (University of Southern California) to work on the neural correlates of risk prediction. He is now chief scientist in the Laboratory for Decision-Making under Uncertainty at the Swiss Federal Institute of Technology, Lausanne (EPFL). The goal of the lab, headed by the Prof. Peter Bossaerts, is to study human decision, in particular, when faced with financial risks.
Research Project
“Should one believe in God?” To answer this question, Blaise Pascal (1623-1662) used expected value theory. According to this theory, the value of a decision equals the expected value (mean) of the associated payoff. Because believing in God gives an infinite gain (Heaven) if God exists and a small loss if it doesn't, the expected value for believing in God is infinite. According to Pascal's wager, it is thus rational to bet on the existence of God. Here is a more mundane question: “Do you prefer a lottery that offers 10 Frs for sure or a lottery that offers 50% chance to win 20 Frs (nothing otherwise)?” The two lotteries have the same expected value (10 Frs), thus if humans follow the expected value theory when taking decision, they will be indifferent. However, humans generally prefer the sure gamble. In order to explain this sensitivity to risk, Daniel Bernoulli (1700-1782) formulated the Expected Utility theory: The value of a decision equals the expected value of the associated utility. When the function linking payoff to utility is concave, the expected utility of the sure gamble will be higher than the risky one and will be preferred. More recently, Harry Markovitz (born in 1927) has formulated the Mean-Variance theory for portfolio selection. In this theory, the value of a prospect is a linear combination of the payoff expected value and variance (a measure of dispersion). Here risk aversion is not explained by a concave utility function but by a penalty imposed on payoff variance.
Many articles have examined the conditions under which Mean-Variance and Expected Utility models coincide, focusing on the shape of the utility function or subtle measures of risk such as semi-variance. There is yet a fundamental difference in the two approaches: Expected Utility requires the explicit learning of probabilities using, for instance, Bayesian updating, with the drawback that, as the number of state probabilities increases, accurate estimation of probabilities becomes impossible (for a lottery “X% chance to win 20 Frs, nothing otherwise” there is only two states: Win and No-Win; and only one state probability to estimate: X). This learning limitation does not hold for the Mean-Variance optimizer, who can simply learn the mean and variance using a reinforcement learning algorithm (reinforcement learning can estimate the mean and variance of a payoff series, 20 Frs, 0 Frs, 0 Frs..., without any reference to states). Taking advantage of this fundamental difference, the aim of this project is to study whether humans decisions are best modeled by Mean-Variance or an Expected-Utility. To do so, we will use Functional Magnetic Resonance Imaging to record brain activity while participants complete a decision-making task involving monetary risk. This task is unique in the sense that we manipulate experimentally the number of states and the link between states and payoffs. Results of this neuroeconomics study will permit to test directly the validity of Expected Utility and Mean-Variance theories, two models that are competing in the economics and finance literature for many years.
Funding
The fact that this study was chosen by the AXA research fund gives an important scientific validation to the project. It also increases the visibility of the project within and outside the academic world. The financial support offered by the Post-Doctoral grant is very welcome because researchers in neuroeconomics live all around the world and key conferences are held each year in the USA and in Japan. It is thus necessary to travel in order to stay in contact with the international scientific community. One particularity of our methodology is to pay participants in function of their performance. This makes their choices more realistic, and allow us to generate financial risk. The grant will allow us to pay participants for their performance. Finally, the use of Functional Magnetic Resonance Imaging (combined with eyes tracking) makes the study relatively expensive.
To add or modify information on this page, please contact us at the following address: community.research@axa.com
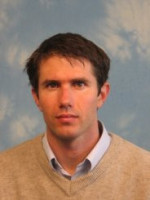
Mathieu
D'ACREMONT
Institution
École Polytechnique Fédérale de Lausanne
Country
Switzerland
Nationality
Swiss
Related articles
Economics
Climate Change
Post-Doctoral Fellowship
Italy
2021.08.31
Climate Change, Trade and Inequalities in the Economic Growth Between Regions
Climate Change and Inequalities in the Economic Growth Between Regions: The Role of Trade Patterns Adjustments and Trade Regulations in... Read more
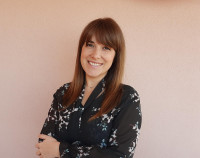
Emilia
LAMONACA
University of Foggia
Economics
Finance
Post-Doctoral Fellowship
Italy
2017.08.31
When misperception of wealth lead to financial crises
Financial literacy : are people equipped to make effective decisions with their money? The first part of his research will... Read more
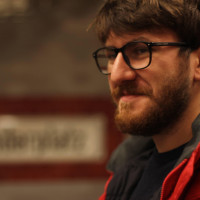
Alberto
CARDACI
Complexity Lab in Economics
Economics
Post-Doctoral Fellowship
France
2017.08.14
Regulating entrepreneurship in the Digital Economy: A French-US comparative approach
Investigating into the world of digital entrepreneurs « One might expect that a strong state would fail to foster innovation... Read more
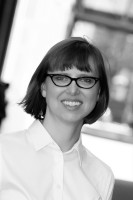
Jen
SCHRADIE