Explainable Artificial Intelligence : a Key Enabler for Using AI in Healthcare and so Expanding Healthcare Access Prof. Thomas Lukasiewicz
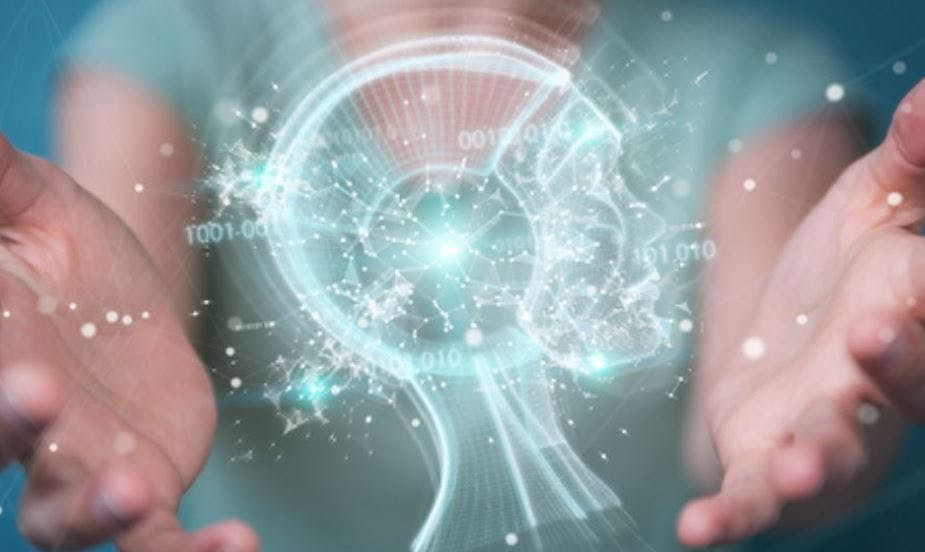
Medical innovation powered by AI
Progress in AI with applications for healthcare began to appear around 2017, when a system was developed that was able to detect skin cancer with the same level of accuracy shown by human experts in the field. Looking more closely at skin cancer detection, we can get an idea of where this progress could take us. There are now research prototypes that are better than human experts at detecting skin cancer. In fact, there are already apps that you can download on your smartphone and use to detect changes in your skin, whether they are skin cancer or not. Obviously, no one should forego expert advice, but these systems aregetting better and better. A number of other milestones have been reached in healthcare. For example, prostate cancer grading by AI is better than human experts, and in 2018, AI systems became better than humans at predicting the 3D structure of proteins. In 2019, they surpassed humans at detecting diabetes-related illnesses in the eye.
The importance of explainability
Despite these advances, being able to explain how they work is a very significant drawback in using neural networks, the technology underlying the current wave of AI systems. Neural networks are extremely powerful, but they are black boxes that turn an input into an output, and we are not able to explain how they work. If we want to make a diagnosis from structured data from the symptoms that a patient has, then we may just return the symptoms that were relevant for the decision as explanation. For example, the diagnosis is flu, and the system may return that thepatient was sneezing, had a headache but had no fatigue. So, that could be returned as an explanation. But there is still no guarantee that the data that is shown is used in a correct way to calculate the outcome. These obstacles are especially valid for healthcare. An incorrect diagnosis of a disease may lead to an incorrect treatment with life-threatening consequences for the patient. We would liket his black box of the neural networks to be explained in such a way that we know that the output is calculated in a correct way from the input.
A way forward for AI healthcare applications
In my AXA Research Fund project, I am building neural-symbolic AI systems that will help us to produce better and less expensive diagnoses, optimize and personalize the treatment of patients, and also preven diseases by collecting and using live data from humans (for example, via wearables) and then predicting the lifestyle and potential risks of these people. This in turn will substantially reduce healthcare costs while improving healthcare availability and reducing mortality and morbidity. We can obtain a more accurate risk prediction, concerning both lifestyle and treatments, and we can also act to reduce our risk. As a side effect of explainability, I also hope to improve the medical understanding of diseases and treatments. The potential of AI for disease prevention, early detection of diseases, better and more affordable diagnosis, and medical decision making in general to designing new pharmaceutical products and optimized treatments can help make healthcare more effective, and also more affordable. Through the use of AI, greater overall medical capacity is available, creating a pathway for expanding healthcare access. For the insurance industry, these benefits will also allow for a more accurate health risk prediction and the possibility for risk reduction- a win/win to create a healthier society.
Discover research projects related to the topic
Financial & Social Inclusion
Finance
Culture & Society
Joint Research Initiative
China
2021.04.19
Understanding the Financial Lives of Low Income Households in China
Leveraging financial diaries research methodology, this joint initiative aims to provide actionable insights about the financial lives of low-income households... Read more
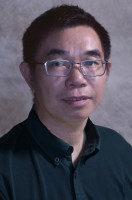
Xiugen
MO