Source: The Conversation
We are currently witnessing a rapidly growing adoption of artificial intelligence (AI) in our everyday lives, which has the potential to translate into a variety of societal changes, including improvements to economy, better living conditions, easier access to education, well-being, and entertainment. Such a much anticipated future, however, is tainted with issues related to privacy, explainability, accountability, to name a few, that constitute a threat to the smooth adoption of AI, and which are at the center of various debates in the media.
A perhaps more worrying aspect is related to the fact that current AI technologies are completely unsustainable, and unless we act quickly, this will become the major obstacle to the wide adoption of artificial intelligence in society.
AI and Bayesian machine learning
But before diving into the issues of sustainability of AI, what is AI? AI aims at building artificial agents capable of sensing and reasoning about their environment, and ultimately learning by interacting with it. Machine Learning (ML) is an essential component of AI, which makes it possible to establish correlations and causal relationships among variables of interest from data and prior knowledge of the processes characterizing the agent’s environment.
For example, in life sciences, ML can be helpful to determine the relationship between grey matter volume and the progression of Alzheimer disease, whereas in environmental sciences it can be useful to estimate the effect of CO2 emissions on climate. One key aspect of some ML techniques, in particular Bayesian ML, is the possibility to do this by account for the uncertainty due to the lack of knowledge of the system, or the fact that a finite amount of data is available.
Such uncertainty is of fundamental importance in decision making when the cost associated with different outcomes is unbalanced. A couple of examples of domains where AI can be of tremendous help include a variety of medical scenarios (e.g., diagnosis, prognosis, personalised treatment), environmental sciences (e.g., climate, earthquake/tsunami), and policy making (e.g., traffic, tackling social inequality).
Unsustainable AI
Recent spectacular advances in ML have contributed to an unprecedented boost of interest in AI, which has triggered huge amounts of private funding into the domain (Google, Facebook, Amazon, Microsoft, OpenAI). All this is pushing the research in the field, but it is somehow disregarding its impact on the environment. The energy consumption of current computing devices is growing at an uncontrolled pace. It is estimated that within the next ten years the power consumption of computing devices will reach 60% of the total amount of energy that will be produced, and this will become completely unsustainable by 2040.
Recent studies show that the ICT industry today is generating approximately 2% of global CO₂ emissions, comparable to the worldwide aviation industry, but the sharp growth curve forecast for ICT-based emissions is truly alarming and far outpaces aviation. Because ML and AI are fast growing ICT disciplines, this is a worrying perspective. Recent studies show that the carbon footprint of training a famous ML model, called auto-encoder, can pollute as much as five cars in their lifetime.
If, in order to create better living conditions and improve our estimation of risk, we are impacting the environment to such a wide extent, we are bound to fail. What can we do to radically change this?
Let there be light
Transistor-based solutions to this problem are starting to appear. Google developed the Tensor Processing Unit (TPU) and made it available in 2018. TPUs offer much lower power consumption than GPUs and CPUs per unit of computation. But can we break away from transistor-based technology for computing with lower power and perhaps faster? The answer is yes! In the last couple of years, there have been attempts to exploit light for fast and low-power computations. Such solutions are somewhat rigid in the design of the hardware and are suitable for specific ML models, e.g., neural networks.
Interestingly, France is at the forefront in this, with hardware development from private funding and national funding for research to make this revolution a concrete possibility. The French company LightOn has recently developed a novel optics-based device, which they named Optical Processing Unit (OPU).
In practice, OPUs perform a specific operation, which is a linear transformation of input vectors followed by a nonlinear transformation. Interestingly, this is done in hardware exploiting the properties of scattering of light, so that in practice these computations happen at the speed of light and with low power consumption. Moreover, it is possible to handle very large matrices (in the order of millions of rows and columns), which would be challenging with CPUs and GPUs. Due to the scattering of light, this linear transformation is the equivalent of a random projection, e.g. the transformation of the input data by a series of random numbers whose distribution can be characterized. Are random projections any useful? Surprisingly yes! A proof-of-concept that this can be useful to scale computations for some ML models (kernel machines, which are alternative to neural networks) has been reported here. Other ML models can also leverage random projections for prediction or change point detection in time series.
We believe this is a remarkable direction to make modern ML scalable and sustainable. The biggest challenge for the future, however, is how to rethink the design and the implementation of Bayesian ML models so as to be able to exploit the computations that OPUs offer. Only now we are starting developing the methodology needed to fully take advantage of this hardware for Bayesian ML. I’ve recently been awarded a French fellowship to make this happen.
It’s fascinating how light and randomness are not only pervasive in nature, they’re also mathematically useful for performing computations that can solve real problems.
July 2019
Prof. Maurizio Filippone
Find more about Maurizio Filippone's project: "Better qualifying uncertainty when studying complex phenoma"
PROJECT PAGE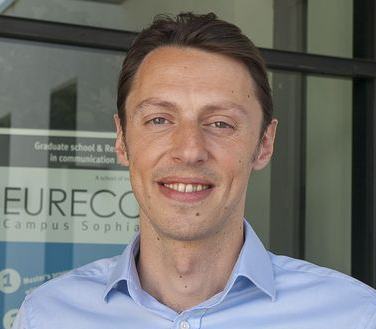
Discover research projects related to the topic
Financial & Social Inclusion
Finance
Culture & Society
Joint Research Initiative
China
2021.04.19
Understanding the Financial Lives of Low Income Households in China
Leveraging financial diaries research methodology, this joint initiative aims to provide actionable insights about the financial lives of low-income households... Read more
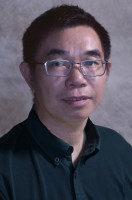
Xiugen
MO